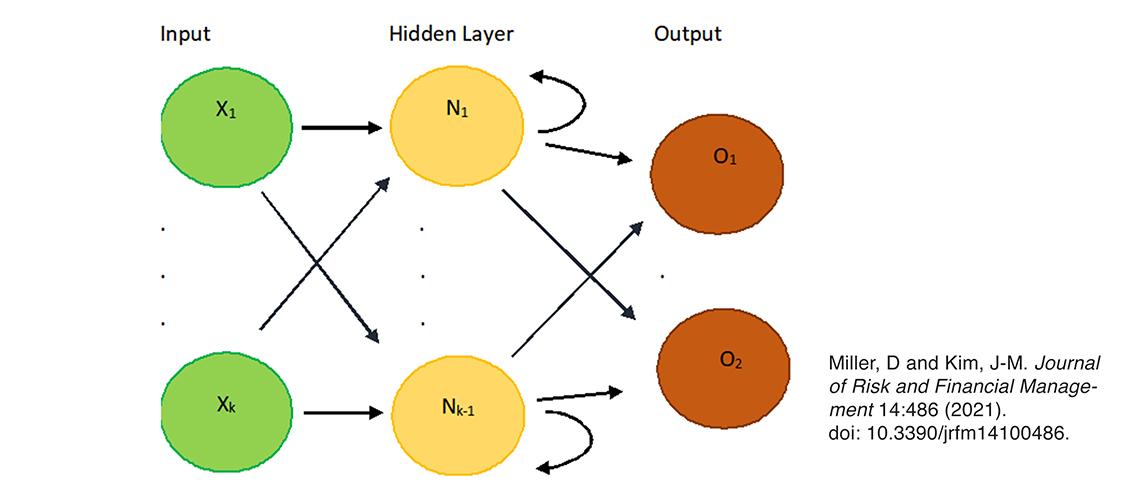
Cryptocurrencies, such as Bitcoin and Dogecoin, have received considerable interest and press coverage since their invention. In a paper published in the Journal of Risk and Financial Management, student Dante Miller, a member of the research group of MSI PI Jong-Min Kim (professor, Science and Math, University of Minnesota Morris), used machine-learning techniques to predict the behavior of the top ten cryptocurrencies. Methods included recurrent neural networks, deep learning neural networks, Holt’s exponential smoothing, autoregressive integrated moving average, ForecastX, and long short-term memory networks. These include both univariate and multivariate models. The results indicate that multivariate models have better prediction accuracy than univariate models. Continued research in this area may eventually show whether these methods could be expanded to other areas such as the stock market or weather. The models were run on MSI’s supercomputers.
The paper can be found on the journal website: Dante Miller and Jong-Min Kim. Univariate and Multivariate Machine Learning Forecasting Models on the Price Returns of Cryptocurrencies. Journal of Risk and Financial Management 14: 486 (2021). doi: 10.3390/jrfm14100486.
Mr. Miller is now attending graduate school at Rice University. A poster about this research, Predicting Cryptocurrency Price Returns Using Machine Leaning Methods, was presented at the 2022 MSI Research Exhibition.
Figure description: Outline for recurrent neural network and long short-term memory network methods. Figure and description, Miller, D and Kim, J-M. Journal of Risk and Financial Management 14: 486 (2021). doi: 10.3390/jrfm14100486.
published on October 18, 2022